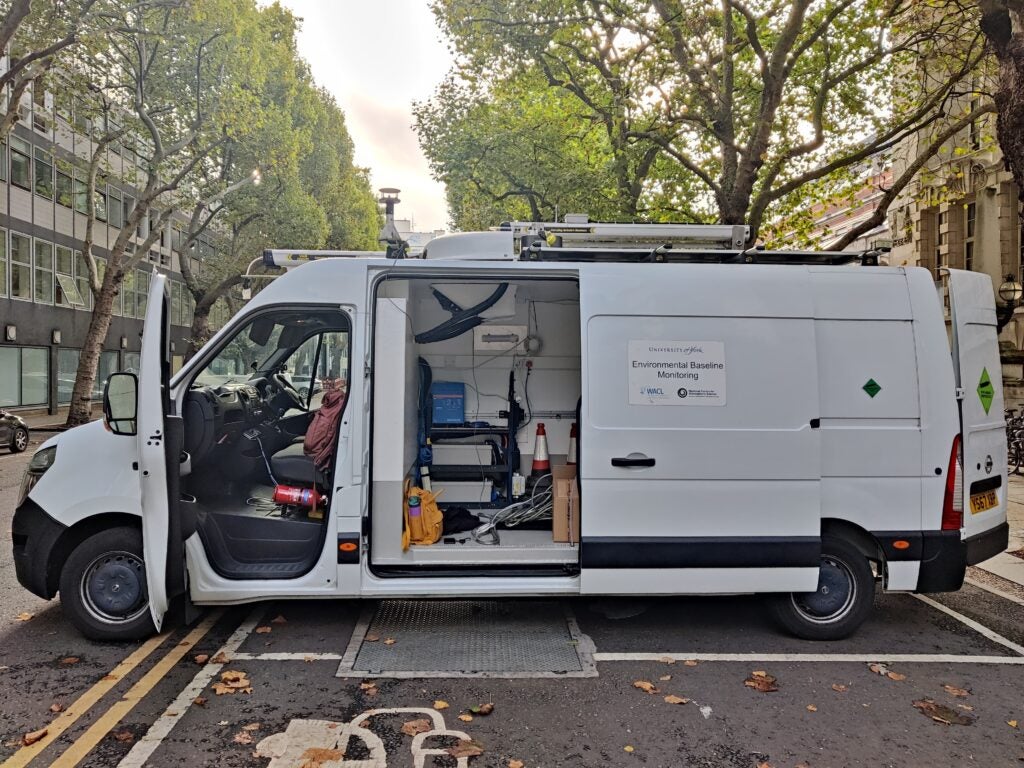
University of York mobile laboratory for measuring ambient air pollution (Photo taken by Shona Wilde).
New study shows just how much congestion intensifies emissions from diesel vehicles
In a recently published study, researchers from the University of York and Environmental Defense Fund show how traffic exacerbates nitrogen oxide (NOx) pollution from road vehicles, in particular from intense emitters like diesel trucks and buses, pointing to solutions that can bring an outsize benefit to air quality.
Comparing pollution with targeted monitoring
EDF and York designed a study that measured air pollution along two distinct routes in the London metropolitan area—one that was extremely congested in Central London and the other farther from the city center where drivers experience more free-flowing conditions including some highway driving. The Central London route was identified as a pollution hotspot in the Breathe London Pilot project, which provided motivation for the new targeted study.
Using York’s mobile monitoring laboratory equipped with fast-response instruments, we collected ambient measurements of both NOx and Carbon Dioxide (CO2) for two weeks along the two different routes to quantify the emission intensity of the London fleet, which included a wide range of vehicle types driving in both heavy congestion and light-traffic conditions. We also used dashboard video recordings to identify which specific vehicle types were the likely cause of hotspots.
Using the latest analysis methods, we mapped the spatial patterns of persistent emission sources, while simultaneously revealing the attributes of the most significant emitters within the vehicle fleet. We found that NOx emissions were a factor of two times higher for fleets with a high proportion of diesel vehicles operating in congested driving conditions, and a factor of five times higher for intense emitters like SCR-retrofit diesel buses and heavy goods vehicles in stop-and-go traffic.
We then compared our data to an existing database of measurements from remote sensors, which measure vehicle emissions more directly at the tailpipe, to verify our ambient data against an established reference. The comparison further demonstrated the congestion effect whereby fleets predominately composed of vehicles rated with the highest emission standards (Euro 6/VI) generated NOx pollution that would be expected from a fleet of lower standard vehicles (Euro 2/II-5/V), when impacted by high traffic.
Our new approach allowed us to focus on the emission intensity of both the overall fleet and specific high-emitting vehicles and make comparisons to established measurements of real-world emissions. The results provided greater insight than standard measurements solely focused on total ambient concentrations.
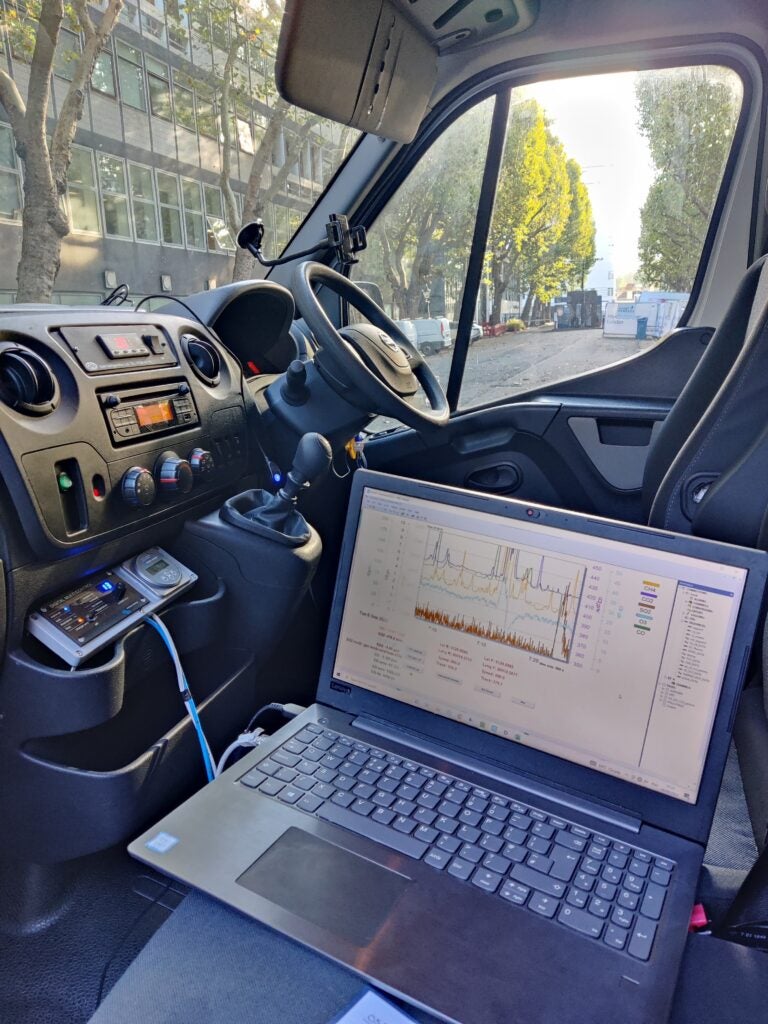
Real-time, high-frequency air pollution measurements. (Photo taken by Shona Wilde)
New methods increase understanding with fewer resources
Beyond illustrating the impact congestion has on emission intensity, the study shows the feasibility of extracting valuable insights from reasonably short mobile monitoring campaigns. Compared to previous large-scale studies, such as the ~1-year long Breathe London mobile study, this campaign was less resource intensive, requiring just two weeks of driving and reducing vehicle and instrument maintenance. This campaign simplified field logistics and increased the efficiency of the data analysis. The new method could also prove useful to scientists and policy makers who want to learn more about local fleet emissions under a variety of traffic conditions in places that aren’t currently well-monitored. Mobile monitoring provided a continuous picture of emissions along a route, not limited to individual locations like stationary monitors.
This approach offers policy makers a new way to spot specific vehicle types and conditions that produce greater emission intensity, so they can develop targeted interventions and monitor progress over time in a cost-effective manner.
While there is no substitute for knowing the exact vehicle emitting pollution, as one might find using remote sensing, this new technique provides useful information in places where remote sensing is either impossible or impractical. It’s also especially useful when exploring the impacts of congestion, as remote sensing is not well suited for stop-and-go traffic.
While the project focused on London, the methods and insights developed can be useful for other cities, particularly those where there are diesel vehicles operating in heavy traffic.
Practical policy applications
Because this method identifies the effects of the highest-polluting vehicles in the most congested areas, it gives local transportation officials a clear roadmap to develop the most impactful solutions.
The adverse effect of congestion on tailpipe emissions can be eliminated with the transition to electric vehicles, which has added benefits for the climate, especially for heavy-duty diesel trucks and buses. Officials could also consider introducing solutions like restricting operating hours for these vehicles in high-traffic areas or creating bus lanes, both of which could ease congestion. The approach could help determine which city-owned vehicles need to come in for maintenance to restore performance of aging exhaust aftertreatment technology.
In cities that already implement clean air zones and technology retrofit programs, air quality can improve even further if high congestion can be better addressed in places where diesel vehicles operate. In London, where the ULEZ has already helped reduce ambient Nitrogen Dioxide (NO2) concentrations, accelerating replacement of the most potent polluters with electric vehicles, starting with the most congested routes, would provide additional climate, health and mobility benefits improving the driving experience for everyone.
To learn more about the study, read the article in Atmospheric Environment: X.